A smart piece of advice passed down the generations is “Don’t put all your eggs in one basket.”; This phrase is the first golden rule of investing. In the event of any lousy day, the crate gets dropped, every one of the eggs will endure the shot at the same time. Henceforth, putting each egg in different bins is comparable to diversification.
Diversification is a technique that reduces risk by dispensing investments among various money related instruments. Under-diversification can lead to “idiosyncratic” risk, and it involves uncertainties and potential problems that are endemic to an individual asset, e.g., the plunge the Macintosh stock took due to Steve Job’s demise.
HUMAN ADVISORY BUSINESS — TAKING THE STAND
Investing and diversifying isn’t as straightforward as it appears, and it involves taking complex decisions. That is the moment when financial advisors or frankly speaking the people with ‘right’ set of knowledge appear in the picture. A financial advisor once depicted his client’s life (his assets) as the many-many pieces of a jigsaw puzzle. The wonderful picture is right there, but it involves the flawless fitting of each piece. They help to complete the picture with a blend of the science of financial planning (using scientific techniques such as Markowitz model) and the art of planning (dealing with individual money mindsets, exploring psychological baggage with money and dealing with any behavioral blocks). The one-stop arrangement which people find to all their financial problems is appointing a financial advisor.
PITFALLS LYING UNDERNEATH
Be that as it may, history stands as a testament to the fact the financial advisors do have flaws, and certain ones are pretty serious. Also, it is essential to keep in mind just how awful the track record of human advisors really is. To start with, at an aggregate level, they are too costly for the general mass to afford. Why would you take the pain to cut your very own lawn, clean your own home, and save through all these years? And then finally turn around and pay thousands per year to have your money being foreseen. Additionally, for firms, charges have not declined because, as the standard item became less expensive, clients were pushed into higher expense items.
Second, the conflicts of interest are pervasive in the industry. The collaboration between human advisors and customers is like a business exchange, in which the advisor has a motivation to augment individual motivators that may vary from investors best interests. The controversy regarding the fiduciary ruling of the ‘client’s best interest law’ clearly poises the fact that the best interest of clients isn’t served at large. E.g., Broker clients dispense more dollars to higher fee funds, and participants tend to perform better when they do not have access to brokers.
Third, Mullainathan et al. (2012) documents that advisers fail to de-bias their clients and frequently reinforce biases that are to their greatest advantage. Advisers encourage returns- chasing behavior and push for actively managed funds that have higher fees, even if the customer begins with a well-expanded, low-charged portfolio.
Fourth, often advisors adopt a one size fits all methodology highlighting a genuine disparity between the client’s risk ability and the portfolio’s actual reality.
To wrap things up, even these advisors are people, and the human mind has a cognitive limit with regards to managing multifaceted problems.
ENTRY OF THE BACKSTAGE PLAYERS – ROBOADVISORS
Consider the possibility that we get a single click solution to the issues stated above. With the advent of technology in our lives, an engaging arrangement can be seen right out from the pocket of fintech. This is where “robo-advisors” take an entry. The exact imagination of them can be deceptive. Truly, there isn’t a genuine robot physically present to guide you in decision making. Rather, it describes online platforms that track investment trends, follow automated algorithms, and recommend opportunities.
We can decipher the robo-adviser as an approach to simplify the set of decisions investors have to make to rebalance their portfolios. When investors have no access to the tool, rebalancing involves a mind-boggling set of choices. They face the daunting task of choosing from many securities and allocating their wealth among the chosen stocks.
Although “robo-advisors” sounds like something out of “Terminator”, there’s nothing so indifferent or frightening about these technology-based financial players.
ANTI-INCUMBENCY OR THE INCUMBENT SURGE?
D’Acunto et al. (2019) suggest that incumbents and new contestants may pursue various methodologies when entering the robo-advising space, in light of economic incentives.
Incumbent players have an existing base of customers to whom they need to cater. Introducing a disruptive product is difficult for them, as it could have severe effects on their customer base. Losing a chunk of revenue in fees could be only one of a handful couple of problematic effects due to the introduction of robo advising for them. But where Incumbents truly lead is the fact that they already have skilled employees they can deploy on the new products. As a result, they tend to offer additional services to their clients, such as hybrid forms of human and robo- advising. New entrants have greater adaptability when presenting innovative products because their strategy is to acquire market share by pulling in new clients to financial advisory or drawing in existing clients incumbents serve. New participants have the spirit of switching things around in the business by alluring propositions such as lower fees, innovative services like tax- loss harvesting, automated execution of decisions but they surely do lack on the infrastructure front and thus would offer fewer support services to their clients.
The robo-advising industry was started as a disruptive play by new entrants. These providers saw robo-advising as an opportunity to help customers human advisers would not serve. The new entrants opened the gates of financial advice to a plethora of users. The broad reach of Robo- advisors helped add age groups and income groups which were unheard to the industry incumbents.
Traditionally the High net worth individuals and ultra-high net worth individuals were only considered to have an appetite for personalized financial advice. But now with the tech-savvy generation, a greater number of people tend to adopt robo-advice. Some major key players in 2017, e.g., Betterment, Wealthfront, and Personal Capital had assets under management (AUM) of about $12 billion, $9 billion, and $4 billion, respectively, from about 306,000, 171,000, and 11,300 customers. Far under 10% of these clients are high-net-worth individuals.
BUILDING BLOCKS OF THE ADVISORY
According to Jung et al. (2019), the working of an RA can be broken down to a five-step or five processes approach
- Asset universe selection –Creating a representative set of assets.
- Investor profile identification – Online surveys & questionnaires concentrated on distinguishing customers’ risk resistance as well as investment targets and horizon. Ordinarily, the inquiries are assembled to comprehend the target risk tolerance through data on age, pay, reserve funds, past speculation experience, and venture objectives.
- Asset allocation/portfolio optimization – This is the major focus or the heart of Robo- advisors, which runs as well as differentiates them. The greater part of the frameworks apply the Modern Portfolio Theory approach), enhanced and adjusted by various methods (e.g., Black– Litterman, VaR, and CVaR optimization).
- Monitoring and rebalancing – Threshold and Calendar rebalancing were the ones referred. Threshold rebalancing involved an automated portfolio adjustment so that the risk is balanced. While Calendar rebalancing is the most straightforward alternative, involving a periodical rebalancing of the portfolio from time to time, varying from months to years.
- Performance review and reporting – While an online site to screen the profits and portfolios was the most mainstream option; certain Robo-advisors had applications and other mediums to notify about their performance.
ETFs — ARE THEY THE RIGHT PIECE OF PIE?
The robo-advising tool deployed in D’Acunto et al. (2019) is a “Portfolio optimizer” focusing on Indian equities and has a target group of individual investors of a large brokerage house in India. Likewise, a striking perception during the observation time frame was the eagerness of Indian financial specialists to pursue the traditional rules of thumb while portfolio selection. Subsequently, individual stocks were viewed as the most loved decision, and the investors were incredulous about ETFs. This particular finding is in accordance with the general trend showing that mutual funds and ETFs account for less than 5% and 0.07%, respectively, of the aggregate stock market.
The goal of automation and technology is greater precision. The use of ETFs will change as Robo-advisors develop and learn more up to date and better systems. So they can invest in a wide cluster of funds, both ETFs and individual securities.
Some imperative highlights of the research in D’Acunto et al. (2019) incorporate the fact that all investors in the sample space had just been acclimated with human financial advice. This is in line with the finding that technological progress in large part is due to the adoption of technology, not just their creation.
THE PERKS OF ADOPTING THE BOT
Robo-advising simplifies the process because automated execution lets investors actualize the advice they receive. Robo-advisors have been around long enough that question is no longer whether you should surrender your investment decisions over to a computer. The question at present is: Why won’t you?
To begin with, they typically use replicable algorithms based on the financial theory, which stands to have a firm footing while designing the portfolios.
Second, they employ technology that in numerous cases, can simplify and accelerate contact with clients. For instance, robo-advisors can push out alerts to customers rapidly in light of news or market changes.
Third, Transparency: Top purposes behind picking them incorporate convenience, straightforwardness, and not being pushed into items clients figure they do not need. Additionally, they expel the awkwardness part which could arise if you don’t like your advisor.
Fourth, an additional advantage of robo-advisers is the greater efficiency in implementing strategies due to built-in automated algorithms. There is no scope of subjectivity.
Fifth, with the steerage in the hands of robo, the odds of an unintended mistake will approach least.
MAJOR FINDINGS of D’Acunto et al. (2019)
USER BEHAVIOR – ADOPTION VS NON-ADOPTION
Initially, utilizing the data, it was confirmed that both the classes (clients and non-clients) are similar and invariant across the demographic characteristics such as age, gender & account age.
Second, Portfolio optimizer invited a higher login rate. Thus, highlighting the fact adopters were more attentive to their accounts. It additionally brought about a higher volume of trade and along these lines, higher trading expenses. Two patterns emerged, first users had a substantially higher AUM and held a larger number of stocks than non-users.
Third, came the uplifting news for Robo-advisors, with the users outperforming non-users over the sample. They had a fair amount of better market-adjusted return.
Better performance is linked with better portfolios hence accurately diversified and a lesser incidence of behavioral biases.
Diversification — Ex-Ante & Ex-Post
Diversification outcomes are judged on two parameters – the number of stocks held and volatility of their portfolios.
Investors who held only 1 or 2 stocks before the optimizer’s use had the dire need to diversify their portfolio. They expanded the number of stocks they held considerably after the utilization of the optimizer. The optimizer accomplished a stunning increment of 100% by and large. The impact was sure notwithstanding for those holding somewhere in the range of 3 and 5 stocks and somewhere in the range of 6 and 10 stocks, however, the evaluated extents of the change declined substantially for the higher number of stocks held. At long last, the change wound up negative and noteworthy for those holding in excess of 10 stocks, which is reliable with the thought that the optimizer may have recommended disinvesting from stocks that ought to be shorted due to the absence of short-selling constraint.
Three speculations convey the outcomes:
To start with, human advisors might be oblivious of the notion of diversification and might themselves show behavioral predispositions that they move to their customers while exhorting them.
Second, diversifying the portfolio involves approving multiple trades in multiple stocks. This very procedure is complicated and conveys its own set of problems, as customers are probably going to have concerns in regard to the nature of each trade and the soundness of each trade. The dialog can end up protracted and rapidly lead to paralysis of trade.
Rather, following the counsel of the robo-advisor is simple and direct as it includes a tick on a box in the wake of seeing the proposed portfolio assignment.
Third, even in the wake of monitoring the upsides of diversification and the fact that investors show behavioral biases, human advisors may choose to cook their recommendation to the flavors of their clients as opposed to amending their mistakes and helping them improve their performance.
The investigation in D’Acunto et al. (2019) identifies a lot of well-recorded inclinations credited to individual investors by prior research. From one perspective, trades suggested by the robo- advising tool ought not to reflect any behavioral biases. While on the other hand, because investors trade more after using the robo-advising tool, the effects of behavioral biases could be higher if investors increased the number of trades, they placed without a direct recommendation by the robo-adviser.
The authors center this examination around on three well-documented behavioral biases, that is, (i) the disposition effect (ii) trend-chasing, (iii) the rank effect. In terms of both disposition and trend-chasing, the users of the optimizer showed an improvement, but in terms of the rank effect, there was no significant change.
PITFALLS — FORESEEN OR UNFORESEEN
Yet we know “All that glitter isn’t gold”; so even after believing robo-advisors can be ultra- beneficial, they do have faults.
Irreconcilable circumstances: There exist conflicts of interest on two conceptual levels: right off the bat, concerning the affiliated brokers, and furthermore concerning the selection of recommended assets. For instance, a few organizations do utilize merchants in their decision, requesting a more expensive rate than ideal for the client (Fein 2015). This demonstrates that a share of the more expensive rate is kept as a benefit by the robo-advisor. Especially the case with the zero-fees robo-advisors.Irreconcilable circumstances: There exist conflicts of interest on two conceptual levels: right off the bat, concerning the affiliated brokers, and furthermore concerning the selection of recommended assets. For instance, a few organizations do utilize merchants in their decision, requesting a more expensive rate than ideal for the client (Fein 2015). This demonstrates that a share of the more expensive rate is kept as a benefit by the robo-advisor. Especially the case with the zero-fees robo-advisors.
Insufficient assessment of risk tolerance and absence of personalization. A commonly expressed point of criticism alludes to the risk appraisal of robo-advisors dependent on prepared questionnaires. The goal-based investing or the humane necessity to gauge differences based on various factors while allocation and rebalancing is a huge concern.
Cocca (2016) shares this view, evaluating the effortlessness of risk tolerance questionnaires as ‘natural’ limit in the development of robo-advice.
No up close and personal contact: Despite the way that the investing business may seem to have every one of their motives about money making, it probably isn’t true in every case.E.g., in wealth management where traditionally high net worth clients are present, personal contact seems to be more critical. For example, a client may be more willing to discuss the consequences of the unexpected passing away of their wife when the long-time human advisor of the family addresses this sensitive issue with due care.
Although they are alike to human advisors in front of the law and play the game by the same rules, Fein (2016) states that robo-advisors just limitedly consider individual client conditions or other external factors, which can lead to fatal consequences, for instance, in plunging market situations.
So robo-advisors will have issues, but there is so much room for improvement that it ought to be easier for them to do better, on average, than human-advisors. Humans are good at maintaining plausible deniability, and in the case of financial advising, that is a severe problem.
References:
Cocca, T. (2016). Potential and limitations of virtual advice in wealth management. Journal of Financial Transformation, 44(1).
Fein, M. L. (2015). Robo-advisors: A closer look. Available at SSRN 2658701.
Fein, M. L. (2016). FINRA’s Report on Robo-Advisors: Fiduciary Implications. Available at SSRN 2768295.
Jung, D., Glaser, F., &Köpplin, W. (2019). Robo-Advisory: Opportunities and Risks for the Future of Financial Advisory. In Advances in Consulting Research (pp. 405-427). Springer, Cham.
Mullainathan, S., Noeth, M., &Schoar, A. (2012). The market for financial advice: An audit study (No. w17929). National Bureau of Economic Research.
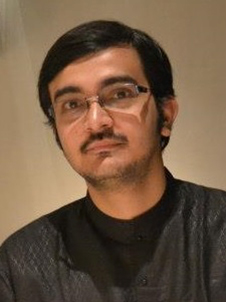
Prof. SamarpanNawn
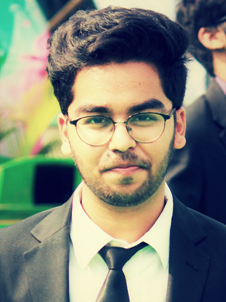
Lakshraj Doshi
Leave a Reply